The Ugly Truth About AI that No One Wants to Hear
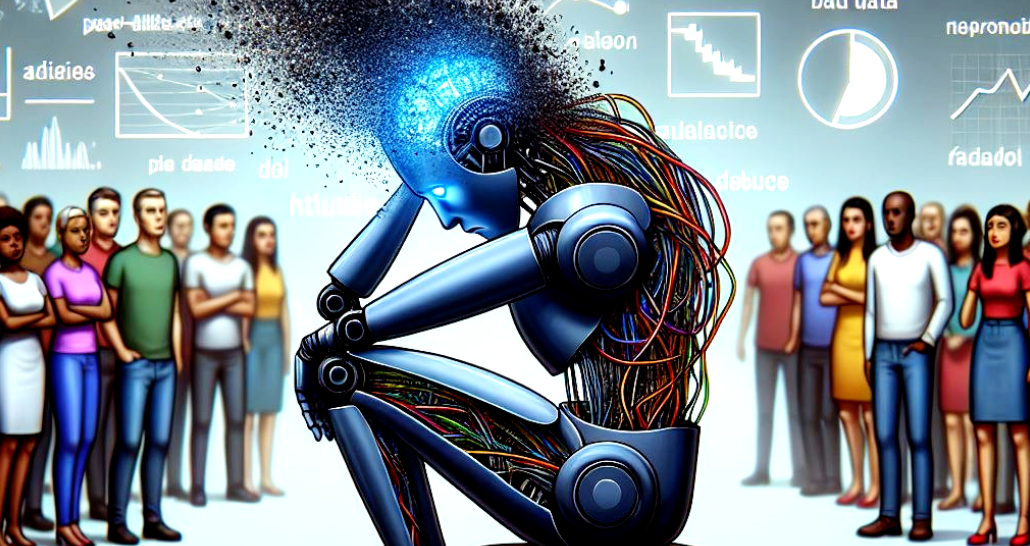
In the rapidly evolving world of artificial intelligence, organizations are racing to implement the latest models and secure their AI systems. However, many are overlooking the most fundamental challenge: data quality. While AI models continue to evolve at breakneck speed, the foundational issue of data quality remains constant and critical to address first.
The Data Quality Imperative
The saying “garbage in, garbage out” has never been more relevant than in the context of AI. High-quality data is the bedrock upon which successful AI implementations are built. When we feed AI systems poor-quality data, it’s like giving them junk food – it might satisfy immediate needs but won’t support the long-term health and effectiveness of the system.
Consider these sobering examples:
· A 2017 self-driving car accident in Florida occurred because inaccurate image annotations prevented the detection of a white truck against a bright sky, resulting in a fatal collision
· Amazon had to withdraw its AI-based recruitment tool because it showed bias against female candidates, having been trained primarily on data from male-dominated resumes
· Microsoft’s AI chatbot Tay became notorious for making offensive comments on social media due to poor data quality in its training data
These failures weren’t due to model selection or security issues – they stemmed directly from data quality problems.
Why Data Quality Matters More Than Model Selection
While organizations often focus on selecting the latest AI models, the reality is that even the most sophisticated algorithms cannot overcome fundamental data quality issues:
· Accuracy and reliability: AI models trained on inaccurate or incomplete data will produce unreliable outcomes, regardless of the algorithm’s sophistication.
· Bias mitigation: Ensuring data quality means addressing biases present in the data, which is essential to avoid perpetuating and amplifying these biases in AI-generated outputs.
· Generalization capability: A diverse and representative dataset enhances an AI model’s ability to perform well across different situations and contexts.
As Andrew Ng, Professor of AI at Stanford University, emphasized: “If 80 percent of our work is data preparation, then ensuring data quality is the most critical task for a machine learning team.”
Have concerns about how your data quality is impacting your AI initiatives? Let’s Discuss!