The AI Efficiency Trap: How Increased Efficiency Drives Greater Demand
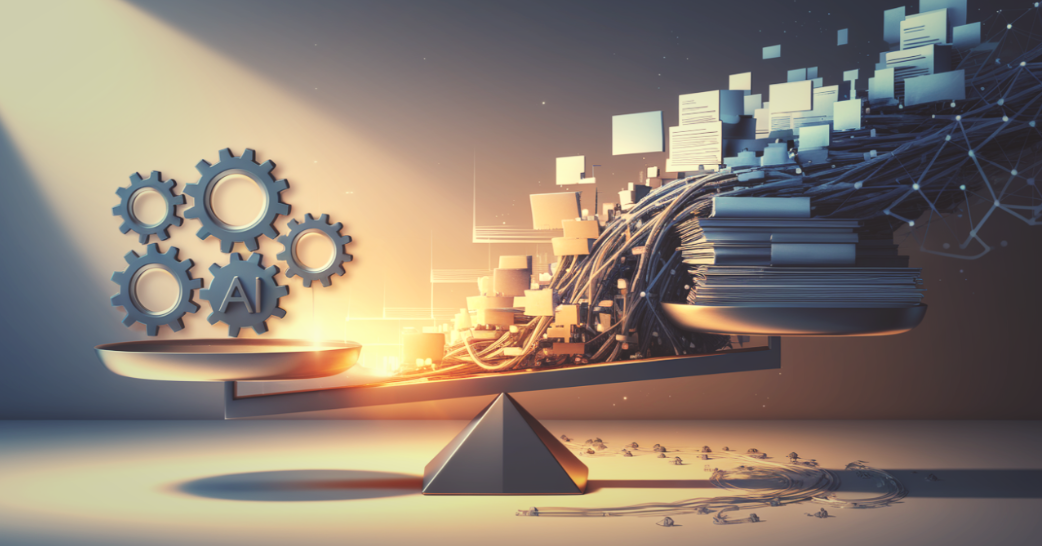
An article by Scott Weiner, NeuEon CTO, AI and Transformation Lead
AI promised to lighten our workloads, but many businesses are drowning in more tasks than ever. Why? The culprit might be AI’s efficiency itself, ironically driving up demand in ways we never anticipated. This head-scratching phenomenon has its roots in a 150-year-old economic paradox that’s more relevant today than ever.
This phenomenon, first identified by economist William Stanley Jevons during the Industrial Revolution, revealed that increased efficiency in coal use led to higher overall consumption due to increased economic activity. Jevons observed that as coal became cheaper and easier to use, industries expanded, which drove demand even higher. We see this paradox in our daily lives: more efficient light bulbs don’t always lower electricity use, and more fuel-efficient cars can lead to increased miles driven.
While AI enhances efficiency by automating tasks and streamlining operations, it can also create new demands for human oversight, data management, and decision-making. This is the Jevons Paradox in action. At NeuEon, we’ve seen firsthand how AI’s efficiency can drive demand, and we help businesses navigate this complexity.
For example, in a 2024 Upwork survey, 77% of employees said AI tools had actually increased their workload. Nearly 39% spent more time reviewing AI-generated content, and 23% had to invest more time learning how to use AI tools effectively.
Industry-Specific Examples of the Jevons Paradox in AI Adoption
This paradox plays out across industries, each with its own version of AI-driven demand. Here are some examples how this paradox is affecting specific sectors, from healthcare to customer service:
1. Healthcare: Faster Diagnostics, More Data
Despite massive technological advances in healthcare over the past 50 years, demand for services has paradoxically skyrocketed. AI-driven diagnostics and tools like robotic surgery have revolutionized healthcare, but they’ve also unleashed a torrent of data requiring more analysis, follow-ups, and oversight, ultimately leading to more sophisticated, data-intensive care rather than reducing workload.
2. Manufacturing: Optimized Processes, Increased Oversight
While AI has streamlined manufacturing with precision robotics and predictive algorithms, it has paradoxically increased workloads for some manufacturers. The flood of data from AI-driven systems requires sophisticated human oversight for interpretation, quality control, and ethical considerations, transforming the nature of expertise needed on the factory floor.
3. Customer Experience (CX): Faster Responses, Higher Expectations
AI-powered chatbots and predictive analytics have streamlined customer service. However, this has raised customer expectations, leading to more complex inquiries that require human agents with specialized skills to handle issues beyond AI’s capabilities.
Of Course, AI Can Reduce Workload Successfully
While the Jevons Paradox explains how increased efficiency often leads to higher demand, it doesn’t always play out that way. It depends on various factors, including the specific type of task being automated, the industry context, and how the AI solution is integrated. In some cases, AI has successfully reduced workloads without adding significant complexity.
For example, in administrative tasks, AI-powered tools for automating data entry, scheduling, and invoice processing have proven to be time-saving and low-maintenance. These tools reduce tedious work without generating additional demand for oversight. Many companies have found that their teams can refocus on higher-value tasks as AI takes over routine processes.
Customer Service Automation: Streamlined Support
Some organizations have implemented AI chatbots that handle the majority of customer inquiries—up to 70% of routine questions—allowing human agents to focus on only the most complex cases. By effectively automating simple tasks, these companies have managed to reduce workloads without creating additional demands for human oversight.
AI in Small Businesses: Efficiency Without Complexity
For small businesses, AI tools like automated marketing platforms and customer relationship management (CRM) systems have streamlined operations without adding new layers of complexity. These systems enable lean teams to manage marketing, customer follow-up, and sales pipelines more effectively, all while keeping operations simple. In these cases, the Jevons Paradoxdoesn’t apply because the AI systems are designed to enhance, not burden, small teams.
It should be noted, it’s an overstatement to say they do so without adding any new layers of complexity. The need for human oversight, potential regulatory challenges, and the possibility of expanded operations due to increased efficiency suggest that some aspects of the Jevons Paradox may still apply, even if to a lesser degree than in other circumstances.
Why the Jevons Paradox Matters for Business Leaders
Understanding the Jevons Paradox is important for business leaders adopting AI. Here’s why:
Strategic AI Adoption
Without a clear plan, AI can increase complexity rather than reduce it. Leaders must ensure that AI truly complements human work.
Is AI enhancing your team’s productivity, or is it adding to their workload?
Increased Human Involvement
Even as AI automates tasks, it often increases the need for human oversight.
Is your team equipped to handle the complexities AI introduces, or could they risk burnout?
Training and Governance
As AI introduces new layers of complexity, continuous training and solid governance frameworks are critical for success.
Do you have the necessary governance and training programs to manage AI’s evolving demands?
Concrete Solutions for Managing AI Complexity
While the Jevons Paradox explains why efficiency gains can lead to more work, here are ways to manage it effectively:
Selective AI Deployment
Use AI where it adds clear value without unnecessary complexity, such as automating repetitive tasks like scheduling.
Governance and Policy Development
Develop AI policies that align with your business goals and risk management strategies.
Ongoing Training and Collaboration
Train your teams to work with AI effectively, ensuring it enhances human work, not replaces it.
Phased AI Implementation
Start small and scale AI projects as they prove their value, allowing teams to adapt without becoming overwhelmed.
Looking Ahead: How AI’s Evolution Could Change the Jevons Paradox
Leaders need to consider how AI’s increased autonomy and advancements like Explainable AI (XAI) will reshape efficiency and accountability. The future of AI will require not only optimized processes but also smarter, more transparent systems. In future articles, we’ll explore how AI’s growing autonomy could reduce or increase the demand for human involvement.
Is your organization ready to navigate AI’s complexity? In upcoming articles, we’ll discuss how AI’s advancements will impact businesses and what leaders need to do to prepare. NeuEon can help guide your AI adoption to ensure long-term success.