AI’s Identity Crisis: Adapting to Radical Changes from Supreme Court Shifts to Scientific Breakthroughs
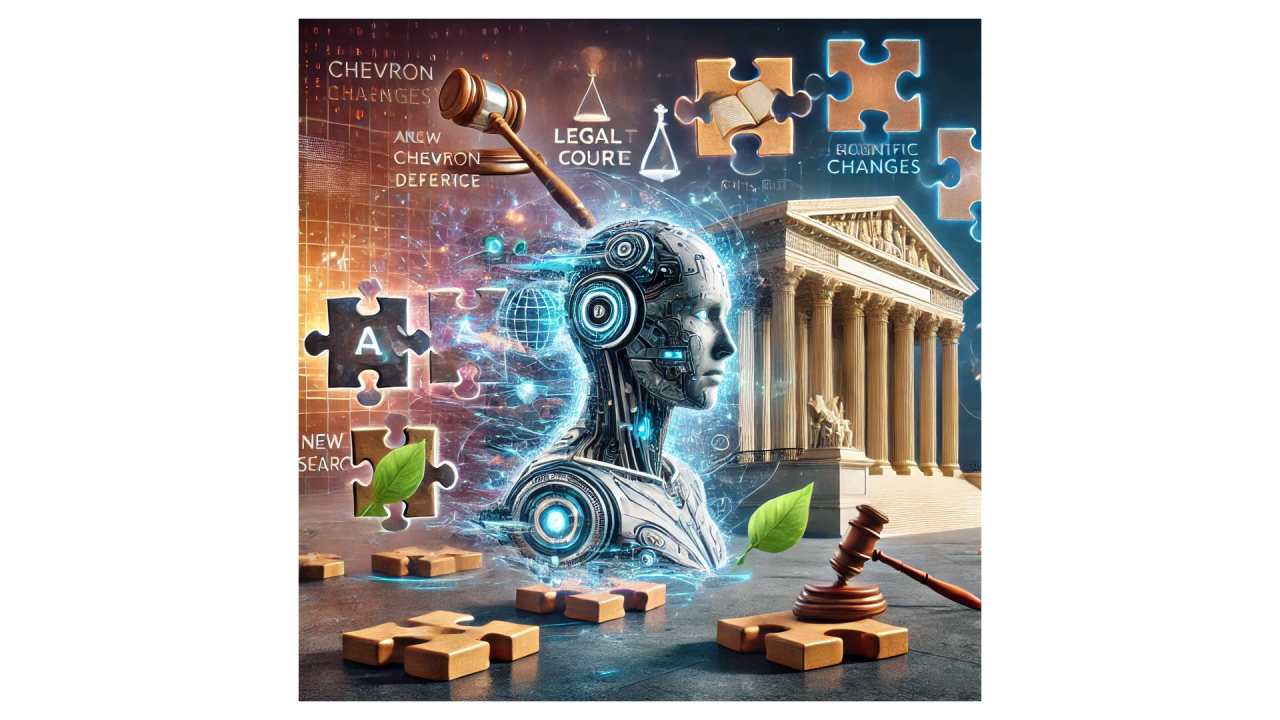
An article by Scott Weiner, NeuEon CTO, AI and Transformation Lead
The transformative impact of Artificial intelligence (AI) across various industries cannot be overstated. Yet, the dynamic nature of knowledge and societal norms presents a formidable obstacle: keeping AI models up-to-date.
Consider the recent Supreme Court ruling which overturned a 40-year-old legal rule called “Chevron deference.” This rule, established in 1984, allowed courts to accept federal agencies’ interpretations of unclear laws if they seemed reasonable. This doctrine allowed agencies like the EPA, OSHA, and the NLRB to wield significant power in shaping regulations across various sectors, from environmental protection to workplace safety. The rationale was that these agencies possessed the expertise to make informed decisions in complex policy areas. This doctrine has been mentioned and used in many legal arguments for decades.
However, the Supreme Court’s ruling in Loper Bright Enterprises v. Raimondo on June 28, 2024, dismantled this long standing precedent. This means from now on, judges do not need to defer to government experts when considering rulings and this will drastically change legal arguments effective immediately going forward. This will change how a huge number of cases are decided from now on.
The problem is that AI models used in the legal field were trained on decades of data that included many references to this now-overturned rule. This means:
- A lot of the information these AI models “know” about how courts interpret laws is now outdated.
- If lawyers or judges use these AI tools, they might get incorrect or irrelevant information based on the old rule.
- This sudden change highlights how quickly AI models can become unreliable when laws or important precedents change.
Challenges in Keeping AI Models Up-to-Date
AI models, especially those based on machine learning, depend on extensive training data. The data forms the bedrock upon which these models learn and make decisions.
However, the ever-evolving nature of knowledge means that what was once accurate can quickly become outdated.
For generative AI, which creates new content based on its training data, this obsolescence can lead to significant issues, such as generating content based on outdated laws, scientific theories, or social norms.
Specific Challenges for Generative AI
Generative AI faces unique challenges due to its reliance on large-scale datasets that span diverse domains. The sheer volume of data makes it difficult to identify and update outdated information. Additionally, generative models can inadvertently perpetuate outdated or incorrect knowledge if not regularly retrained.
Large Language Models (LLM) are basically statistic engines, they keep track of the relationships between words and concepts and so if something is mentioned many times it tends to carry greater weight in the decision process
For instance, a generative AI model trained on legal texts that include the now-overturned Chevron precedent might continue to reference it, leading to inaccuracies in legal decision-making.
Impact of Outdated Training Data on AI Performance
Outdated training data can compromise the performance and credibility of AI models. In the legal domain, reliance on defunct precedents can result in flawed legal advice or judgments.
This problem is not limited to legal issues however. In scientific research, for example, AI models might base their predictions or analyses on superseded theories, leading to incorrect conclusions. This is not limited to LLMs and can happen in any sector, for example in 2021 with Zillow’s home-buying algorithm. The company’s AI-powered model, which was used to estimate home values and make purchase offers, failed to accurately predict rapid market changes during the COVID-19 pandemic. This led to Zillow overpaying for properties and ultimately resulted in the company shutting down its home-buying business, laying off 25% of its workforce, and writing down hundreds of millions of dollars in losses. The failure of Zillow’s algorithm can be attributed to what’s known in machine learning as “concept drift,” where the relationship between input variables and the target variable (home prices) changed rapidly, and the model failed to adapt.
This series of events highlights the challenges of relying on AI models for high-stakes business decisions, especially in rapidly changing market conditions. Zillow’s experience serves as a cautionary tale about the limitations of AI in predicting complex, real-world phenomena like housing markets without further advancements in the technology.
Case Study: Supreme Court Overturning Chevron
The Chevron U.S.A., Inc. v. Natural Resources Defense Council, Inc. decision, established in 1984, has been a cornerstone of administrative law in the United States.
In June 2024, the Supreme Court overturned the Chevron precedent, fundamentally changing how courts interpret federal agency regulations. This landmark decision has significant implications for the legal landscape, affecting countless legal cases and documents.
Consequences of the Chevron Decision Being Defunct
AI models in the legal domain are trained on extensive datasets comprising legal texts, case laws, and judicial opinions. These models use precedents like Chevron to interpret laws, generate arguments, and provide legal advice. The overturning of Chevron presents a unique challenge as these models must now disregard a precedent that has been a foundational element of their training.
The removal of Chevron from the legal framework creates a gap in the training data of legal AI models. These models must adapt to the new legal context without relying on the now-invalid precedent. Failure to do so could lead to erroneous legal interpretations and advice, undermining trust in AI-driven legal solutions.
Organizations relying on foundational models like OpenAI’s GPT 4o model must wait for OpenAI to refresh their model and hope they “clean” it sufficiently of the reliance on the legal work related to the Chevron doctrine. However in the meantime what do they do? Also realize this problem is not isolated to legal issues. It can be found in every area where changes in fundamental knowledge may have a massive effect and more subtle changes in fact patterns may as well.
For example discovery of new scientific breakthroughs, or massive economic changes like during Covid outbreak where businesses saw demand radically change and supply chain forecasting became unstable.
Ethical Considerations in AI Model Updates
Updating AI models isn’t just a technical challenge; it also raises ethical considerations:
Transparency: Organizations must be transparent about when and how their AI models are updated, especially in high-stakes applications such as healthcare or criminal justice.
Fairness: Updates should be monitored to ensure they don’t introduce or exacerbate biases against certain groups.
Accountability: Clear protocols should be established for who is responsible when updated models make errors or cause harm.
Privacy: When updating models with new data, organizations must ensure they’re not violating individual privacy rights.
Consent: In some cases, it may be necessary to obtain consent from users before applying updated models to their data or decisions affecting them.
Addressing ethical concerns is necessary for maintaining public trust in AI systems and ensuring responsible development and deployment.
Advanced Strategies for Keeping AI Models Current
Fortunately foundation models and internal models at an organization can be (and should be) updated frequently.
Continuous updates to AI models are required to ensure they reflect the latest knowledge and standards. This process involves not only updating the training data but also retraining the models and validating their outputs.
Regular updates help maintain the accuracy and reliability of AI systems, ensuring they provide relevant and correct information.
Using advanced techniques this process can be optimized to minimize time and resources needed.
Leveraging Advanced Techniques to Address Dependencies on Third-Party Models
For many businesses, leveraging third-party AI models like OpenAI’s GPT-4 or Google’s Gemini offers a fast track to sophisticated AI capabilities without the need to build models from scratch. However, this reliance also introduces dependencies on the release cycles and updates of these external providers. Here are some strategic steps businesses can take to mitigate the risks associated with such dependencies and ensure they remain agile in a rapidly evolving AI landscape.
Implement Retrieval-Augmented Generation (RAG): RAG systems combine the generative capabilities of language models with real-time information retrieval, ensuring that AI outputs are based on the most current data available. This approach enhances the relevance and accuracy of AI-generated content.
Custom Knowledge Bases: Businesses can create custom knowledge bases that are regularly updated with the latest legal precedents, scientific advancements, or other critical information. These knowledge bases can augment the generative capabilities of third-party models.
Continuous Model Monitoring: Companies should implement robust monitoring systems to detect any degradation in model accuracy over time, comparing the model’s current performance against baseline metrics.
Data Freshness and Labeling: It’s a good idea to use first-party data that is up-to-date and well-labeled, maintaining data privacy and reducing the risk of outdated or inaccurate information.
Human-in-the-Loop Approach: Maintaining human oversight in AI systems allows for real-time verification and correction of AI-generated responses, especially in domains where accuracy is critical, such as legal advice.
Regular Testing and Validation: Organizations should conduct frequent testing of their AI systems against the most current information available, creating test sets that reflect recent changes in the field and validating the model’s responses against these updated benchmarks.
Hybrid Approaches: Combining AI models with traditional rule-based systems for certain applications can provide a safety net for areas where AI models might lag in updating their knowledge.
Prompt Engineering: Developing sophisticated prompts that include instructions to consider the most recent information or to flag potential outdated responses can help mitigate issues with model currency. For example, when using ChatGPT you might instruct the LLM to ignore any legal document citing Chevron… However this is no guarantee not to mention hallucinations are still an issue.
Strong prompt engineering skills are needed to effectively use large language models and maximize their potential. This is not about just explaining what you want more clearly, it’s a whole set of techniques and strategies that require a deeper understanding of how these LLM actually work.
NeuEon has a whole workshop on more advanced prompt strategies because this is so necessary today. In the future there may be advancements that make this less necessary but right now, if you use an LLM and don’t have strong prompt engineering skills chances are you are not getting the best, most reliable and helpful responses from an LLM. This translates into risk, efficiency and quality issues.
Collaborative Ecosystems: Engaging in collaborative ecosystems with other organizations and researchers can help in quickly identifying and addressing accuracy issues across different domains.
Be cautious
It’s important to note that while these strategies can significantly improve the accuracy of responses, they may not completely eliminate the risk of outdated information, especially in rapidly changing fields.
Disclaimers: So it is a good idea for companies to maintain a cautious approach and consider implementing disclaimers or warnings about the potential for outdated information, particularly for critical applications.
Employee Training: Companies should also invest in ongoing training and education for their AI teams to ensure they are applying the most current methodologies for maintaining AI accuracy and reliability.
Model Editing Techniques
Model editing techniques are methods designed to modify specific knowledge or behaviors in pre-trained machine learning models, particularly large language models, without full retraining.
Think about it — we’ve got these incredibly complex models, like GPT or LLaMA, and we’re figuring out ways to update them on the fly. It’s not just about keeping them current; it’s about addressing those thorny issues of bias and inaccuracy that can creep in.
While model editing techniques for LLMs show promise and are attracting interest from major tech companies, they are still primarily in the experimental and research phase.
Current model editing techniques for LLMs face several significant challenges. While these methods aim to improve specific aspects of an LLM’s knowledge or performance, they often result in unintended consequences. The primary issues include degradation of the model’s general abilities, overfitting to edited facts, excessive alterations to model weights, and difficulty in balancing edit accuracy with minimizing drawdown on unrelated tasks. There are other issues as well. Still, these strategies are worth knowing about because directionally we likely need ways to be more surgical in our updates if we want to maintain up-to-date models.
Here are some of the approaches being worked on:
Locate-then-Edit Methods
There are a few approaches we’re seeing in this space. One that’s particularly interesting is what is called “locate-then-edit” methods. It’s a bit like finding a specific memory in a human brain and then carefully adjusting it.
First, we use techniques like causal tracing to pinpoint where certain information is stored in the model’s vast network of parameters. Then, we make precise edits, often using what’s known as rank-one updates to the model weights. It’s delicate work, aiming to change specific knowledge without messing up the model’s other capabilities.
Meta-Learning Methods
Another approach that’s gaining traction is meta-learning. This is about teaching models to “learn how to learn” — a concept that I find deeply philosophical. It’s like we’re instilling a form of cognitive flexibility in these AI systems, allowing them to adapt quickly to new tasks with minimal data. Some methods focus on learning optimal initialization parameters for quick fine-tuning, while others involve neural networks with memory capabilities that can rapidly acquire and apply new knowledge.
Post-Edit Models
We’re also seeing interesting developments in post-edit models. This is where we refine AI-generated outputs, like machine translations, through automated techniques. It’s a way of fine-tuning the model’s performance on specific tasks, focusing on areas where it’s shown weaknesses or biases.
Memory-based Methods
Memory-based methods for editing language models use external storage to keep updated information, rather than directly changing the model’s parameters. Key examples include Semi-Parametric Editing with a Retrieval-Augmented Counterfactual (SERAC) model and Graph Memory-based Editing for Large Language Models (GMeLLo). These approaches offer advantages like preserving original model knowledge, better scalability for multiple edits, and easier updates.
However, they face challenges in efficient retrieval and seamless integration of stored information. Memory-based methods show promise in balancing model integrity with flexible, scalable knowledge updates.
Fine-Tuning on Specific Datasets
Of course, we can’t forget about fine-tuning. This is where we take a pre-trained model and give it additional training on carefully curated, task-specific datasets. It’s like sending an AI back to school for a specialized degree. The beauty of this approach is that it requires less data and computational power than training from scratch, but can still yield significant improvements in performance and relevance.
Explainable AI and Transparency
One aspect that I think is crucial in all of this is explainable AI. Implementing explainable AI techniques can help identify when and why a model might be providing outdated, inaccurate or even biased information. This transparency allows for quicker detection and correction of errors.
As we develop these editing techniques, we need to ensure we understand why and how our models are making decisions. This transparency isn’t just about catching errors; it’s about building trust in these systems that are becoming increasingly integrated into our lives.
What is exciting about model editing techniques is their potential to keep AI systems relevant, accurate, and aligned with human values in a rapidly changing world. It’s a testament to the incredible progress we’re making in AI, but also a reminder of the responsibility we have to guide this technology in a direction that benefits humanity.
Conclusion
Keeping AI models up-to-date is a critical challenge in the rapidly evolving landscape of technology and knowledge. The Chevron deference case study highlights how sudden changes in law can render AI models obsolete overnight, but this issue extends far beyond the legal domain.
Key takeaways:
- Regular updates are necessary for maintaining AI model accuracy and reliability.
- Advanced techniques like RAG, custom knowledge bases, and model editing show promise in addressing the update challenge.
- Ethical considerations must be at the forefront of AI update strategies.
- Human oversight remains an imperative in the update process.
Looking ahead, the field of AI model updating is likely to see significant advancements. We can expect more sophisticated, automated update techniques, better integration of real-time data, and increased focus on explainable AI to ensure transparency in the update process.
As AI continues to play an increasingly important role in our society, We must focus on keeping these systems current, accurate, and ethically sound.
It’s an exciting and challenging frontier in AI research and development, one that will require ongoing collaboration between technologists, ethicists, and domain experts.
NeuEon is here to help
NeuEon understands the challenges your organization faces in navigating AI adoption. With our low-cost, and comprehensive evaluation, we’ll help you confidently chart the right path forward. Ready to discuss your AI strategy? We’re here to assist you anytime. Let’s start the conversation: NeuEon AI Advisory Services.